Amazon Web Services MLS-C01 Exam With Confidence Using Practice Dumps
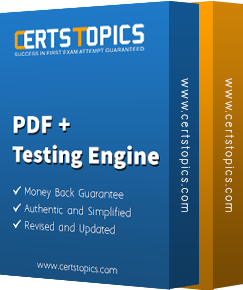
MLS-C01: AWS Certified Specialty Exam 2025 Study Guide Pdf and Test Engine
Are you worried about passing the Amazon Web Services MLS-C01 (AWS Certified Machine Learning - Specialty) exam? Download the most recent Amazon Web Services MLS-C01 braindumps with answers that are 100% real. After downloading the Amazon Web Services MLS-C01 exam dumps training , you can receive 99 days of free updates, making this website one of the best options to save additional money. In order to help you prepare for the Amazon Web Services MLS-C01 exam questions and verified answers by IT certified experts, CertsTopics has put together a complete collection of dumps questions and answers. To help you prepare and pass the Amazon Web Services MLS-C01 exam on your first attempt, we have compiled actual exam questions and their answers.
Our (AWS Certified Machine Learning - Specialty) Study Materials are designed to meet the needs of thousands of candidates globally. A free sample of the CompTIA MLS-C01 test is available at CertsTopics. Before purchasing it, you can also see the Amazon Web Services MLS-C01 practice exam demo.