Databricks Databricks-Generative-AI-Engineer-Associate Exam With Confidence Using Practice Dumps
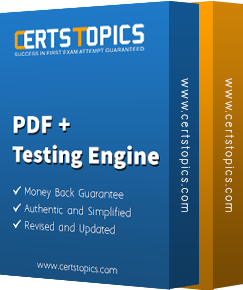
Databricks-Generative-AI-Engineer-Associate: Generative AI Engineer Exam 2025 Study Guide Pdf and Test Engine
Are you worried about passing the Databricks Databricks-Generative-AI-Engineer-Associate (Databricks Certified Generative AI Engineer Associate) exam? Download the most recent Databricks Databricks-Generative-AI-Engineer-Associate braindumps with answers that are 100% real. After downloading the Databricks Databricks-Generative-AI-Engineer-Associate exam dumps training , you can receive 99 days of free updates, making this website one of the best options to save additional money. In order to help you prepare for the Databricks Databricks-Generative-AI-Engineer-Associate exam questions and verified answers by IT certified experts, CertsTopics has put together a complete collection of dumps questions and answers. To help you prepare and pass the Databricks Databricks-Generative-AI-Engineer-Associate exam on your first attempt, we have compiled actual exam questions and their answers.
Our (Databricks Certified Generative AI Engineer Associate) Study Materials are designed to meet the needs of thousands of candidates globally. A free sample of the CompTIA Databricks-Generative-AI-Engineer-Associate test is available at CertsTopics. Before purchasing it, you can also see the Databricks Databricks-Generative-AI-Engineer-Associate practice exam demo.