Snowflake ARA-C01 Exam With Confidence Using Practice Dumps
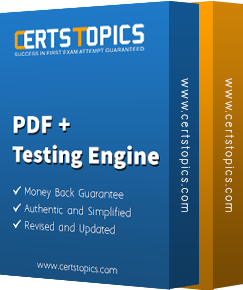
ARA-C01: SnowPro Advanced: Architect Exam 2025 Study Guide Pdf and Test Engine
Are you worried about passing the Snowflake ARA-C01 (SnowPro Advanced: Architect Certification Exam) exam? Download the most recent Snowflake ARA-C01 braindumps with answers that are 100% real. After downloading the Snowflake ARA-C01 exam dumps training , you can receive 99 days of free updates, making this website one of the best options to save additional money. In order to help you prepare for the Snowflake ARA-C01 exam questions and verified answers by IT certified experts, CertsTopics has put together a complete collection of dumps questions and answers. To help you prepare and pass the Snowflake ARA-C01 exam on your first attempt, we have compiled actual exam questions and their answers.
Our (SnowPro Advanced: Architect Certification Exam) Study Materials are designed to meet the needs of thousands of candidates globally. A free sample of the CompTIA ARA-C01 test is available at CertsTopics. Before purchasing it, you can also see the Snowflake ARA-C01 practice exam demo.